The Next Breakthrough: AI Battery Control
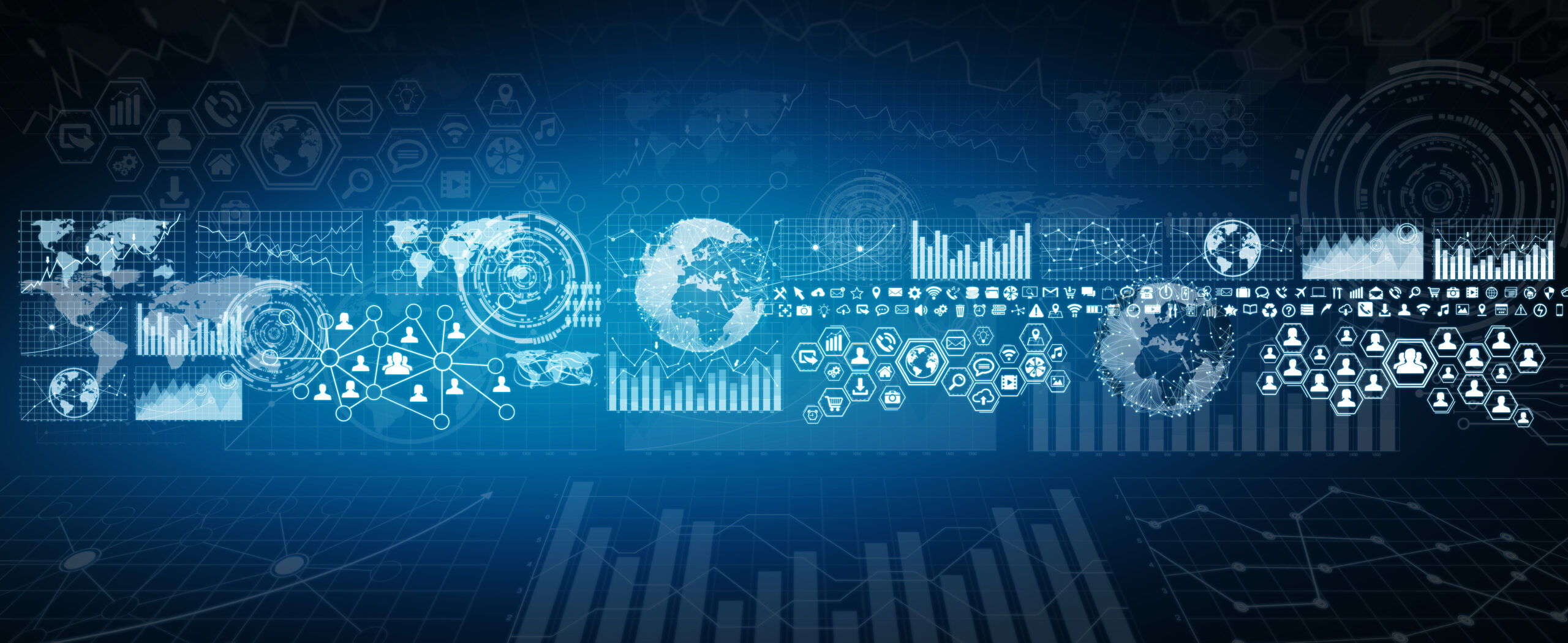
Business Digitals
blogs and media
The next big breakthrough in batteries is not just about new materials or chemical compositions but about intelligent, AI-driven control.
The Next Breakthrough: AI Control of the Battery
In the rapidly evolving landscape of technology, the next significant leap in battery innovation may not stem from advancements in chemistry but from the integration of Artificial Intelligence (AI) in battery management. As we delve into this transformative potential, it becomes evident that data is the cornerstone of AI training and decision-making, directly influencing the performance, accuracy, and reliability of AI models. However, the current battery data, primarily consisting of voltage, temperature, and current, is lacking in the feature richness needed for creating robust machine learning models. This blog post explores how augmenting battery data with Electrochemical Impedance Spectroscopy (EIS) data can revolutionize AI control of batteries, leading to unprecedented improvements in cycle life, charge time, charge density, cost, performance, and safety.
The Importance of Data in AI
Data is the lifeblood of AI. It is the raw material from which AI models learn, make decisions, and improve over time. The quality, quantity, and diversity of data directly impact the effectiveness of AI models. In the context of battery management, traditional data sources such as voltage, temperature, and current provide a limited view of the battery’s state. These parameters, while essential, do not offer a comprehensive picture necessary for advanced AI-driven insights.
Limitations of Current Battery Data
Current battery data is sparse and often insufficient for creating a wide array of features required for sophisticated machine learning models. Voltage, temperature, and current are fundamental parameters, but they do not capture the intricate details of a battery’s internal state and behavior. This limitation restricts the ability of AI models to accurately predict and optimize battery performance, leading to suboptimal outcomes in terms of cycle life, charge time, and overall efficiency.
Challenges in Acquiring Sufficient Data
For machine learning models to be robust, lots of data is required. Getting EIS data at scale is challenging since deploying a commercial off-the-shelf potentiostat in each battery or module is not feasible due to size and cost. To effectively train machine learning models using EIS, it is essential to collect extensive quantities of data across various battery types and models. This can be achieved by leveraging the latest advancements in EIS semiconductor chips, as detailed in the article “From Lab to Field: Scaling EIS Technology with Semiconductor Chips for Battery Systems” ( From Lab to Field: Scaling EIS Technology with Semiconductor Chips for Battery Systems | SigmaSense)
Augmenting Battery Data with EIS
EIS offers a solution to the limitations of current battery data. EIS measures the impedance of a battery at various frequencies, providing a rich set of data that reflects the battery’s internal electrochemical processes. By incorporating EIS data, we can significantly enhance the feature set available for machine learning models, enabling more accurate and comprehensive analysis.
Benefits of EIS Data
AI models trained on augmented data can more accurately predict and manage the battery’s state of health, leading to optimized charging and discharging cycles. This can potentially double the cycle life of batteries, reducing the frequency of replacements and lowering overall costs. With a deeper understanding of the battery’s internal state, AI can optimize charging protocols to reduce charge times without compromising safety or longevity. This is particularly beneficial for applications requiring rapid charging, such as electric vehicles and portable electronics. EIS data can help identify previously unavailable charge densities, allowing for more efficient use of the battery’s capacity. This can lead to longer usage times between charges and improved performance in energy-demanding applications. By optimizing battery performance and extending cycle life, AI-driven battery management can reduce the need for expensive materials and frequent replacements. This not only lowers costs but also has positive environmental implications by reducing waste and resource consumption. AI models leveraging EIS data can fine-tune battery performance to meet specific application requirements, whether it’s maximizing energy density extending cycle life, enhancing power output, or balancing between them. This level of customization can lead to significant advancements in various industries, from consumer electronics to renewable energy storage. Safety is paramount in battery management. EIS data provides detailed insights into the battery’s internal state, enabling AI to detect and mitigate potential safety issues before they escalate. This proactive approach can prevent dangerous situations such as thermal runaway, ensuring safer operation across all applications.
Real-World Applications
The automotive industry stands to benefit immensely from AI-controlled batteries. Extended cycle life and decreased charge times can make EVs more practical and appealing to consumers, accelerating the transition to sustainable transportation. Efficient and reliable energy storage is crucial for the widespread adoption of renewable energy sources. AI-driven battery management can enhance the performance and longevity of storage systems, making renewable energy more viable and cost-effective. From smartphones to laptops, consumer electronics rely heavily on battery performance. AI-controlled batteries can offer longer usage times, faster charging, and improved safety, enhancing the user experience and driving innovation in product design. Industries that rely on battery-powered equipment can benefit from reduced downtime, lower maintenance costs, and improved safety through AI-driven battery management. This can lead to increased productivity and operational efficiency.
Setting New Standards in Safety and Performance
The integration of AI with augmented battery data, particularly EIS, is poised to set new standards in battery safety and performance. By providing a richer set of features for machine learning models, EIS data enables more accurate predictions, optimized management, and proactive safety measures. This holistic approach to battery management can transform how we use and interact with batteries, driving innovation and efficiency across multiple sectors.
Conclusion
The next big breakthrough in batteries is not just about new materials or chemical compositions but about intelligent, AI-driven control. By augmenting traditional battery data with EIS and other advanced parameters, we can unlock a new era of battery performance, safety, and efficiency. This approach not only addresses the limitations of current battery management systems but also paves the way for innovative applications and sustainable solutions across various industries. As we continue to explore the potential of AI in battery management, the future looks bright for a world powered by smarter, more efficient, and safer batteries.